In highly competitive industries like lodging, pricing is key. Even more so, this is true in today’s climate, as travel continues to recover and hotel prices are projected to increase over the next year. With competition at every corner, providing the best rate for your accommodations is essential to securing new bookings.
Finding the optimal rate that both appeals to the consumer and meets your bottom line can be challenging. This is where predictive pricing models can help. Developing the right machine learning (ML) model and having the right data can give you the insight needed to determine the price point that will attract customers and also maximize your sales and profits.
Our hypothesis…
Our data science team looked to test a hypothesis around ML models that lodging companies traditionally use to inform pricing. The hypothesis was that Foursquare Places data could be used to improve the performance of existing pricing models by studying the places surrounding lodging units. Adding such detailed information about the places located near a lodging unit allows you to capture more information about the unit and better establish pricing. For any given unit, Places can provide data points, such as the number of restaurants or coffee shops nearby, whether there is parking available, and how popular and expensive nearby venues are.
We specifically looked to determine whether Foursquare’s Places-based attributes provide value for building pricing prediction models at vacation home rental companies like Airbnb, VRBO and Awaze. We also wanted to find out which Places feature set would be most effective for this type of model.
What we did…
Vacation rental companies use pricing models to provide their users (the property owners who rent out their spaces) with an estimate of how much they should rent a unit for. The models used typically include features like how many rooms a unit has, whether it’s a house or an apartment, how many people it can hold, and what city or neighborhood it’s in. Based on these features – and using historical pricing data – they train an ML model that predicts what the price of a unit should be.
For example, suppose a property owner is renting their unit for the first time and doesn’t know what the price should be. A vacation rental company has a model that essentially looks for similar units based on the set of features mentioned above, and outputs a price. The model has learned what the important features that contribute to the pricing are based on the historical data.
To test our hypothesis, we first built a model to simulate existing pricing models using a publicly available dataset in Kaggle that included information on 74,000 Airbnb units across six U.S. cities including New York, San Francisco, Los Angeles, Boston, Chicago and Washington, D.C. The Airbnb dataset contained information about different Airbnb units, including the number of beds, the type of unit (house, apartment, room), whether there’s an elevator, etc. It also included the nightly rate that each unit was rented for.
Using XGBoost, we then built an almost identical model with the same Airbnb dataset, and then added Places Attributes data. The Places data used included data on the number of venues surrounding each Airbnb unit, as well as features describing these venues. It included data points, such as the number of retail shops, restaurants, and sports venues within a certain distance of the airbnb unit, as well as attributes of the surrounding venues, such as average popularity score and price range.
Lodging companies could have data on the city or neighborhood the venue is in, but our data is more granular because it describes the actual venues in that city/neighborhood, and moreover, the venues close to the Airbnb units, which is more specific than grouping by neighborhood.
The results…
Places data proved to be an effective input for predictive pricing models.
We found that the combined model, which used both Airbnb and Foursquare’s Places features, performed significantly better than the baseline model (which only used Airbnb features). We observed a 6.3% increase in CV r^2 and a 16% decrease on the MSE.
Modeling with Airbnb + Places Features
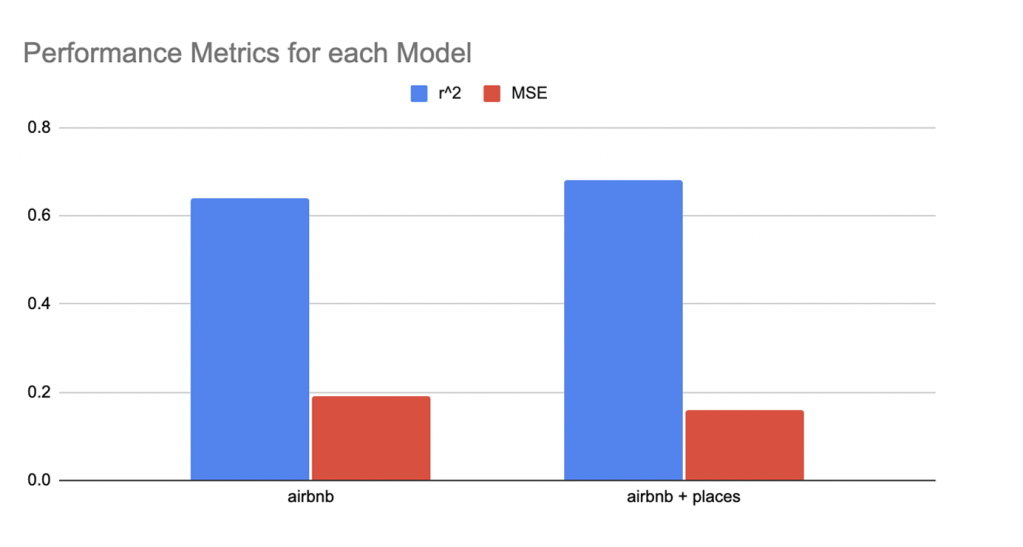
We also studied feature importance of the combined model, and saw that some of the added Places features had high coefficients, indicating that they were good at predicting price, and on occasion were even better than the Airbnb features themselves. Some of the most predictive Places features were (in order of importance):
- Number of sports venues within 1 km of the Airbnb unit
- Number of nearby venues that were labeled as being ‘very expensive’
- Number of lodging venues within 1 km of the Airbnb unit
- Number of healthcare venues within 1km of the Airbnb unit
- Number of cafes within 1 km of the Airbnb unit
- Average popularity score of the surrounding venues
- Number of dining and drinking venues within 1km of the Airbnb unit
- Minimum distance of a safety venue
- Average rating of nearby venues
Feature Importance of Combined Features Model
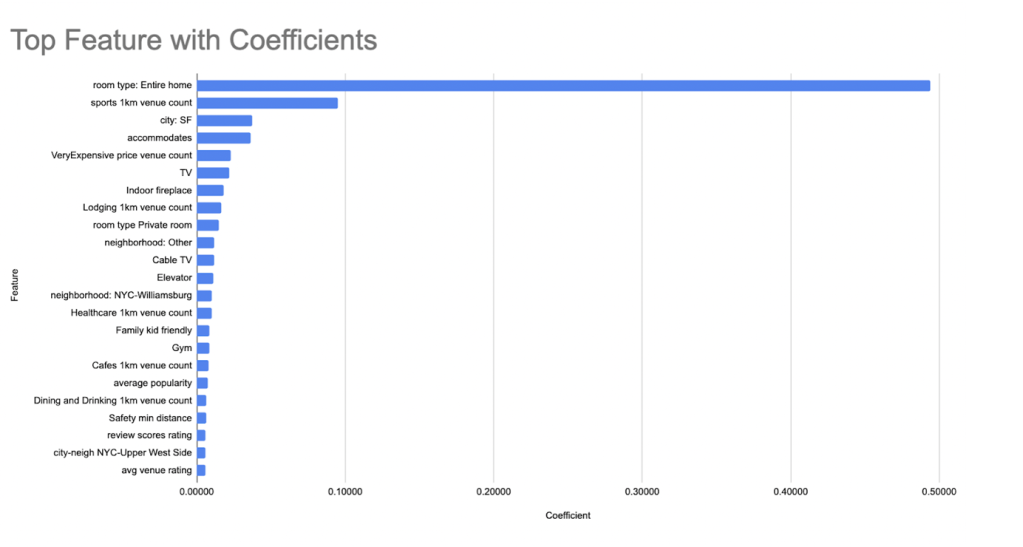
Including Places features in the base pricing model improved its performance, suggesting that pricing of an Airbnb unit is tied to the attributes of the venues around it. In particular, note the features listed above that reference a type of venue “within 1km of the Airbnb unit.” This means that information about venues within 1km of the Airbnb, which is quite a small radius, as well as metrics like venue popularity and venue rating, are important for price prediction.
With the success of this model, our team looks to explore more possibilities to improve predictive analysis as well other ML applications using similar methodologies and Places Attributes data. For example, consider the addition of visits features to the model; this could inform foot traffic data in the area surrounding lodging units, which could also have an impact on pricing.
The model can also help us understand market share and how the price of a lodging business is affected with respect to how many other lodging venues surround it. Or it can aid in site selection, by helping inform what locations are good for pricier rentals.
Want to see how Foursquare’s geospatial technology and data enrichment can help you develop or improve pricing models for your business? Connect with our team to find out.